Build Deeper: The Path to Deep Learning

4.7 out of 5
Language | : | English |
File size | : | 12532 KB |
Text-to-Speech | : | Enabled |
Screen Reader | : | Supported |
Enhanced typesetting | : | Enabled |
X-Ray | : | Enabled |
Print length | : | 273 pages |
Lending | : | Enabled |
Hardcover | : | 124 pages |
Item Weight | : | 10.8 ounces |
Dimensions | : | 5.51 x 0.51 x 8.27 inches |
Deep learning is a subfield of machine learning that uses artificial neural networks to learn from data. Neural networks are inspired by the human brain, and they can be trained to perform a wide variety of tasks, including image recognition, natural language processing, and speech recognition.
History of Deep Learning
The history of deep learning can be traced back to the 1940s, when researchers first began to develop artificial neural networks. However, it was not until the 2000s that deep learning began to gain widespread attention. This was due in part to the development of new algorithms that made it possible to train deep neural networks on large datasets.
Key Concepts of Deep Learning
The key concepts of deep learning include:
- Artificial neural networks: Neural networks are the building blocks of deep learning models. They are composed of layers of interconnected nodes, and they can be trained to learn complex relationships in data.
- Deep learning models: Deep learning models are composed of multiple layers of neural networks. They are typically trained on large datasets, and they can be used to perform a wide variety of tasks.
- Convolutional neural networks (CNNs): CNNs are a type of deep learning model that is specifically designed for image recognition. They are able to learn the hierarchical features of images, and they can be used to achieve state-of-the-art performance on a variety of image recognition tasks.
- Recurrent neural networks (RNNs): RNNs are a type of deep learning model that is designed for processing sequential data. They are able to learn the dependencies between elements in a sequence, and they can be used for a variety of tasks, such as natural language processing and speech recognition.
Applications of Deep Learning
Deep learning has a wide range of applications, including:
- Image recognition: Deep learning models can be used to identify objects in images, and they can be used for a variety of applications, such as facial recognition, object detection, and medical imaging.
- Natural language processing: Deep learning models can be used to process and understand natural language. They can be used for a variety of applications, such as machine translation, text summarization, and question answering.
- Speech recognition: Deep learning models can be used to recognize spoken words. They can be used for a variety of applications, such as voice control, dictation, and customer service.
- Medical diagnosis: Deep learning models can be used to diagnose diseases and predict patient outcomes. They can be used for a variety of applications, such as cancer detection, diabetic retinopathy diagnosis, and heart disease prediction.
Challenges of Building Deep Learning Models
Building deep learning models can be a challenging task. Some of the challenges include:
- Data requirements: Deep learning models require large amounts of data to train. This can be a challenge for tasks where it is difficult to collect enough data.
- Computational requirements: Training deep learning models can be computationally intensive. This can be a challenge for tasks that require real-time performance.
- Overfitting: Deep learning models can be prone to overfitting, which is when the model learns the training data too well and does not generalize well to new data. This can be a challenge to avoid, and it can lead to poor performance on real-world tasks.
Tips for Building Deep Learning Models
Here are some tips for building deep learning models:
- Use a large dataset: The larger the dataset, the better the model will perform. If you do not have access to a large dataset, you can try using data augmentation techniques to increase the size of your dataset.
- Use a powerful computer: Training deep learning models can be computationally intensive. If you do not have access to a powerful computer, you can try using cloud computing services.
- Tune the hyperparameters: The hyperparameters of a deep learning model are the parameters that control the learning process. Tuning the hyperparameters can be a time-consuming process, but it can improve the performance of the model.
- Use regularization techniques: Regularization techniques are used to prevent overfitting. There are a variety of regularization techniques that you can use, and the best technique will depend on the specific task that you are working on.
Deep learning is a powerful tool that can be used to solve a wide variety of problems. However, building deep learning models can be a challenging task. By following the tips in this article, you can increase your chances of success.
4.7 out of 5
Language | : | English |
File size | : | 12532 KB |
Text-to-Speech | : | Enabled |
Screen Reader | : | Supported |
Enhanced typesetting | : | Enabled |
X-Ray | : | Enabled |
Print length | : | 273 pages |
Lending | : | Enabled |
Hardcover | : | 124 pages |
Item Weight | : | 10.8 ounces |
Dimensions | : | 5.51 x 0.51 x 8.27 inches |
Do you want to contribute by writing guest posts on this blog?
Please contact us and send us a resume of previous articles that you have written.
Book
Novel
Chapter
Text
Genre
Reader
Paperback
Sentence
Shelf
Glossary
Bibliography
Foreword
Preface
Codex
Tome
Classics
Library card
Biography
Reference
Dictionary
Character
Librarian
Catalog
Card Catalog
Borrowing
Periodicals
Study
Scholarly
Lending
Reserve
Academic
Journals
Rare Books
Special Collections
Literacy
Thesis
Dissertation
Awards
Reading List
Book Club
Michael J Makley
Ann L Johnson
Alison Howard
Lynne Avril
Jennifer Fletcher
Shelda Sauls
Izzy House
David Pogue
Diane Knoll
Paul A Etoga
Peter Sekirin
Rachel Quinn
Mark Scheppert
Swami Dayananda Saraswati
Simon Carswell
Ali Lowe
Marsha Shapiro
Nigel Thorley
Alfie Kohn
Greg Hutchins
Light bulbAdvertise smarter! Our strategic ad space ensures maximum exposure. Reserve your spot today!
- Emmett MitchellFollow ·2k
- Jan MitchellFollow ·3.1k
- Asher BellFollow ·5.7k
- Edgar HayesFollow ·5.7k
- Marvin HayesFollow ·17.7k
- F. Scott FitzgeraldFollow ·5.5k
- Terry BellFollow ·12.2k
- Eddie PowellFollow ·9.1k
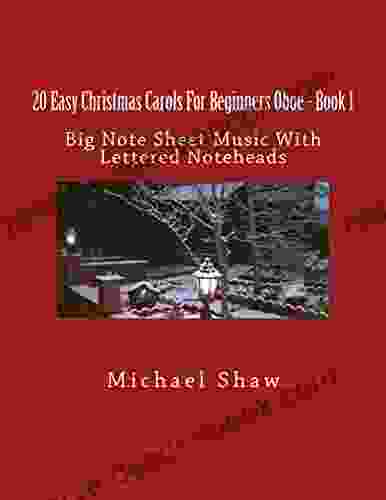

An Immersive Exploration into the World of Big Note Sheet...
: Embarking on a Musical Odyssey The pursuit...
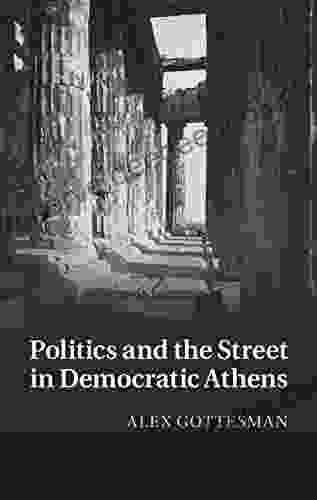

Politics And The Street In Democratic Athens
The streets of democratic Athens...
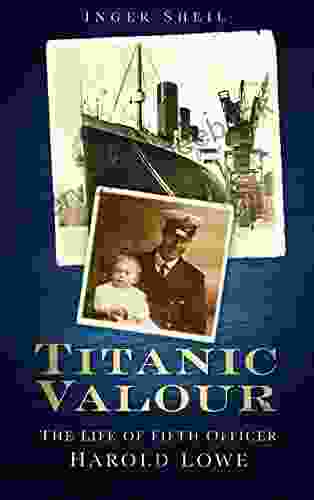

The Extraordinary Life of Fifth Officer Harold Lowe: From...
Harold Godfrey Lowe (21...
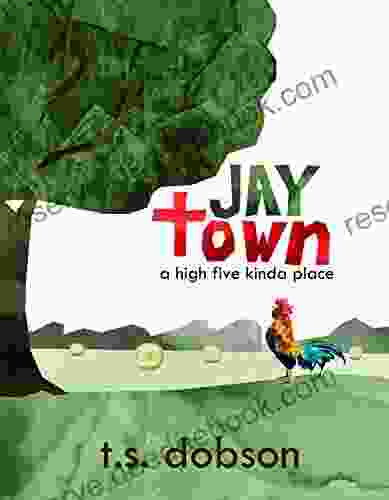

Discover Jay Town: A Place Where High Fives and Community...
Nestled amidst rolling hills and...
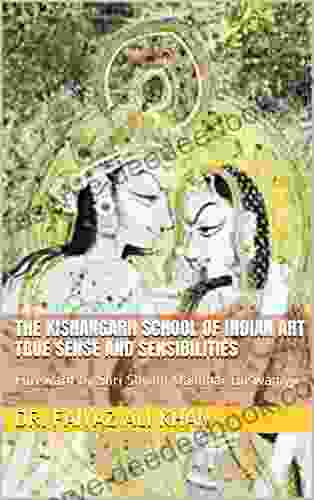

The Kishangarh School Of Indian Art: True Sense And...
Amidst the diverse tapestry of Indian art,...
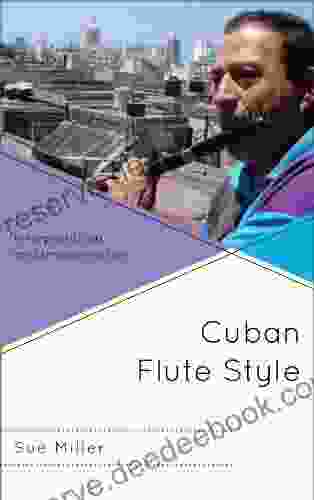

Cuban Flute Style Interpretation and Improvisation: A...
The Cuban flute style is a...
4.7 out of 5
Language | : | English |
File size | : | 12532 KB |
Text-to-Speech | : | Enabled |
Screen Reader | : | Supported |
Enhanced typesetting | : | Enabled |
X-Ray | : | Enabled |
Print length | : | 273 pages |
Lending | : | Enabled |
Hardcover | : | 124 pages |
Item Weight | : | 10.8 ounces |
Dimensions | : | 5.51 x 0.51 x 8.27 inches |